The challenges of developing autonomous vehicle systems aren’t only in the edge cases – a growing body of research continues to highlight that rainfall could be problematic too.
Safety is a cornerstone of autonomous vehicles. Autonomy promises unwavering concentration while navigating the world’s complex, crowded and variable road networks. But while the latest vehicles are using multimodal sensor suites and gaining 360° vision that no human could match, they face some familiar perception challenges in heavy rain.
This is already a problem for production vehicles. Last autumn, the AAA (American Automobile Association) carried out closed-circuit testing of ADAS weather resilience, targeting a roof-mounted high-pressure water jet at the windshield-mounted cameras while driving. The effects were marked; test cars struck a static vehicle target in 33% of runs at 56km/h, while active lane keeping proved ineffective during 69% of runs at 72km/h. None issued warnings that system performance had been compromised.
“Water droplets can influence the ability to spot lane markers and accurately measure the distance to surrounding vehicles, since the camera vision is obscured as rain passes in front of the camera lens,” explains Josh Van Wynsberghe, senior engineer of telematics and data insights at the AAA. “In heavy rain, the vehicle’s windshield wipers can also cause the vehicle to lose track of the lane markers as the wipers temporarily block the camera as it moves by, and wet pavement will also reduce its ability to stop, due to decreased traction.”
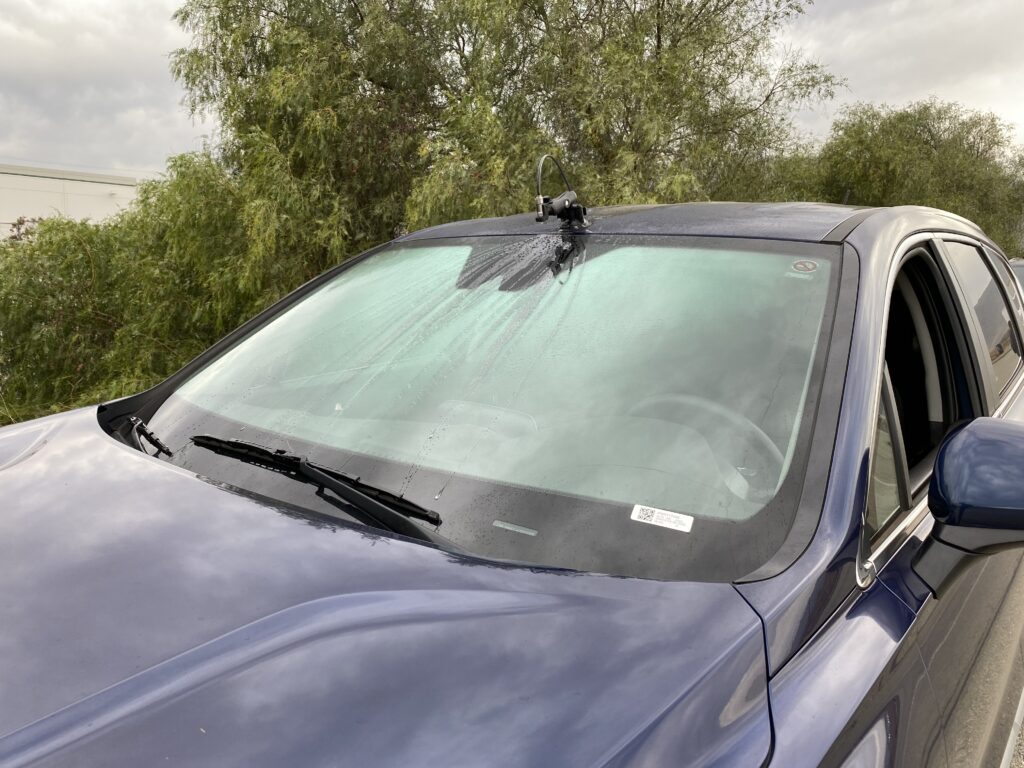
Amazon’s Mobility as a Service subsidiary, Zoox, is also broadening the scope of its testing to cover a wider range of conditions. Its first Level 3 autonomous vehicles were deployed in California’s Bay Area in 2017, focused on AI training for dense urban driving, and the fleet was extended to humid Las Vegas in 2019. Seattle received its first Level 3 Toyota Highlander SUVs last autumn, where the wetter climate will help validate hardware that removes water and debris from the sensors.
Ryan McMichael, senior manager of sensors and systems for advanced hardware at Zoox, believes multimodality is vital. Zoox’s 360° self-driving stack now includes thermal imaging alongside radar, lidar and visible cameras, and is identical to the technology used for its Level 5 robotaxi, which allows that knowledge to be shared.
“We complement real-world driving with tests such as weather chamber and wind tunnel tests, which allow us to precisely characterize the adverse weather problem. Our physical testing is combined with aerodynamic simulations, used to predict airflow and the accumulation of precipitation around the vehicle,” he explains.
“We have also invested in full-stack simulation capabilities that allow us to assess the impact of degraded sensor performance on our perception algorithms, and any potential impact to the safety of the system. All these methods are used together to improve our understanding of the problem and aid our evaluation of solutions.”
From its headquarters in Minnesota, VSI Labs is offering partners opportunities to validate autonomous driving technologies in conditions ranging from heavy winter snow to summer thunderstorms and fog, augmented by data-recorded drives across the breadth of the USA. Founder and president Phil Magney says emerging technologies are beginning to overcome some of the traditional shortfalls.
“RGB cameras – the most common type used for both ADAS and automated driving – can struggle [in heavy rain]. If the human can’t see well, the camera probably can’t either. Thermal cameras are looking for a heat signature, so work well in challenging conditions, and near-infrared cameras also see much better in low light conditions,” he says.
“These are coming – but they’re still relatively expensive, and it’s a nice-to-have, not a must-have. The New Car Assessment Program will likely stimulate demand for thermal cameras, because pedestrian detection in low light conditions is now a criterion. At the moment, you don’t need a thermal camera to get a five-star rating, but that’s about to change. Then volume leads to reduction in cost, and pretty soon it can be part of a typical ADAS package.”
Lidar to the rescue?
Cost reductions are making lidar increasingly viable, too. Ouster has supplied 10,000 of its VCSEL- and SPAD-based systems, with challenging off-road vehicle applications including dusty open pit mines in China and heavy Canadian snow. Its solid-state digital flash units are due for mass-market vehicles from 2025, featuring a new Chronos chip that is claimed to provide improved memory, dynamic range and detection accuracy while cutting costs andenabling easier integration.
“When it comes to autonomous vehicles, sensor redundancy is key,” says Mark Sandoval, VP for automotive products and programs at Ouster. “Each sensor – camera, radar and lidar – has its strengths and weaknesses. Cameras lack depth perception, don’t perform well in inclement weather or night-time conditions and can be easily tricked by light. While radar performs well in all weather and light conditions, its low resolution makes it unreliable at object identification. Lidar experiences significantly less image distortion than the camera in environmental conditions such as rain due to the aperture size, shutter speed and return processing of the sensor. Water doesn’t obscure the lidar signal and range images, even if there are water droplets on the sensor. This, combined with lidar’s high resolution and ability to detect depth, make it a highly reliable perception sensor.”
At the UK’s University of Warwick, the WMG Intelligent Vehicles Group is testing the resilience of lidar in heavy rain, aimed at understanding its advantages and limitations within a perception system. Researchers subjected lidar sensors to several intensities of rain using WMG’s 3xD full-vehicle, driver-in-the-loop CAV simulator, and Dr Valentina Donzella, WMG’s associate professor of intelligent vehicles sensors, says the results suggest lidar’s not infallible either.
“Lidar is affected in a different way [to cameras], because it’s emitting light beams. These can hit a droplet of rain, be reflected back and tell you there is an object there, when it is actually a droplet. So you will start to have a lot of false positives, or noise for the sensor, and some of the light can be absorbed by the rain droplet,” she explains.
“When the rain rate increases, the range of the lidar decreases, so we cannot detect objects that are farther away. So if it were able to detect objects up to 100m away, it will only detect objects that are in close proximity, and the detections will be much noisier, because lots of the beams are reflected by droplets of rain.”
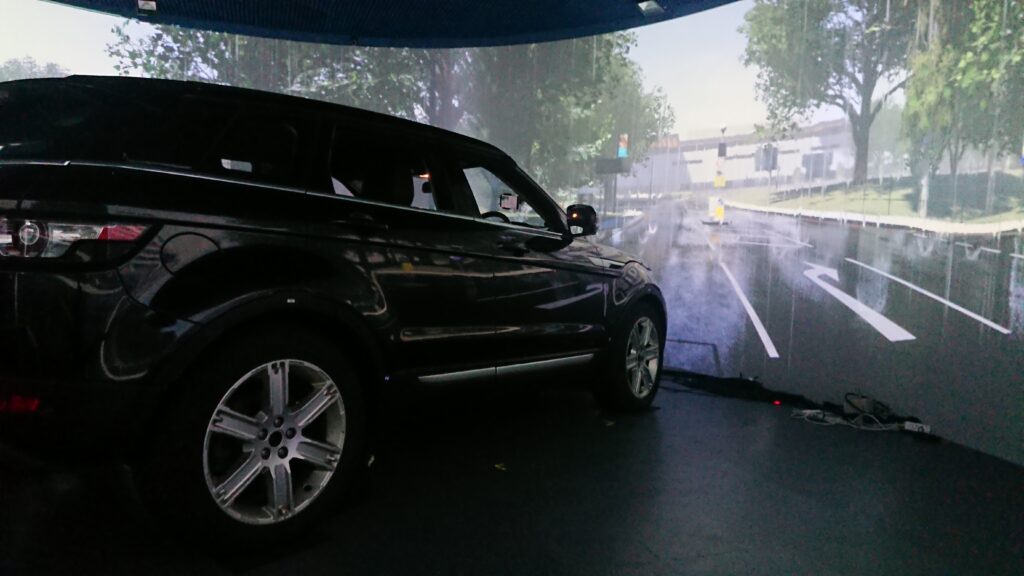
The road to L4
The pan-European AI-SEE project, which is led by Mercedes-Benz and has 20 other industry partners, is also seeking to develop and test – on road and in laboratories – a robust sensor suite for all-weather Level 4 autonomy. With a production target of 2030, this will include new lidar, infrared cameras and 4D MIMO radar sensors, fused using novel AI algorithms and backed up by high-definition mapping to improve localization in poor visibility.
McMichael agrees that high-definition maps will be useful in heavy rain, but advises against overreliance. “The danger in using maps alone without additional data inputs is that maps represent how the world looked at some point in the past,” he comments. “Imagine a road where the lane lines have been repainted. If the map was generated even one day before the construction, then without real-time sensor or perception data, a vehicle relying only on a map would drive on the wrong part of the road.
“Accurately perceiving the world is even more challenging in adverse weather conditions like heavy rain, which only further amplifies the need to have high-quality sensors and a strong perception system.”
In the meantime, perhaps one of the safest responses to adverse weather could be for vehicles to accept the limitations of the technology on board, Donzella says. A more advanced sensor suite would enable vehicles to respond intelligently to poor driving conditions, and exercise caution when perception is compromised.
“At the moment – even combining the different sensor technologies – in heavy rain, when the driver needs to have the most support, the sensors are not able to properly support the understanding of the environment. If we are able to do sensor fusion, where we use partially covered images from the camera with some information from radar or infrared solutions and reduce the speed, we will still be able to support the driver,” she says.
“I think there is a need for industry suppliers, camera suppliers, software suppliers and also academia to work together and overcome these challenges. We are sending robots on the streets with humans inside – we want to make sure that they are safe in all the possible conditions,” she concludes.
Get a grip
Although it’s less frequent on a global basis, snow presents sizeable challenges for perception systems, according to VSI Labs’ Phil Magney. It can not only obscure the field of vision, hide lane lines and dazzle traditional cameras with high-intensity light reflections, but can also result in ice build-up on sensor apertures. Lidar and thermal cameras can’t be fitted behind UV-protected windscreens, which means their field-of-view isn’t being kept clear by wiper blades.
Even in heavy snow, the company’s testing has shown that positioning technology can be a resilient backup, offering virtual lane lines for vehicles to follow. However, high-level autonomous driving systems would have to respond to more than just a reduction in visibility, Magney adds.
“The other big factor that comes into play is a loss of grip,” he says. “Just as a human driver may slow down quite a bit when it gets slippery, an automated system has to do the same thing – and that also applies to ADAS. You need to have algorithms that can sense these conditions and adjust output accordingly.
This feature was first published in the April 2022 edition of Autonomous Vehicle International. You can subscribe to receive future issues, for free, by filling out this simple form.